Stochastic Gradient Descent Methods with Biased Estimators
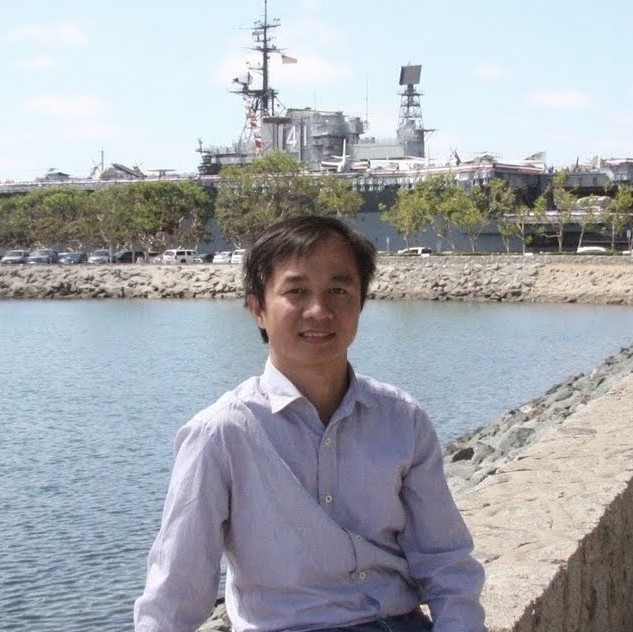
Quoc Tran-Dinh is currently with the Department of Statistics and Operations Research, The University of North Carolina at Chapel Hill, USA. Previously, he was a faculty member at Faculty of Mathematics, Mechanics, and Informatics, VNU University of Science in Hanoi. He obtained his Bachelor and MSc from Vietnam National University in Hanoi, and his Ph.D. from Department of Electrical Engineering and Optimization in Engineering Center, KU Leuven, Belgium. He was a postdoctoral researcher at Ecole Polytechnique Federale de Lausanne (EPFL), Switzerland, before joining UNC-Chapel Hill in 2015. His research mainly focuses on numerical methods for continuous optimization, including convex, nonconvex, and stochastic optimization and related problems. He currently serves as an associate editor of the Computational Optimization and Applications (COAP) and Mathematical Programming Computation (MPC) journals.
The gradient descent algorithm is perhaps one of the most popular methods in practice, especially in machine learning. In the last two decades, research on this topic has become extremely active, leading to various algorithms and interesting theoretical results. In the first part of this talk, I will take an opportunity to briefly discuss some recent progress on this topic, including algorithms, variants, and practical and theoretical aspects. In the second part of my talk, I will present some of our recent results on stochastic gradient-based methods for large-scale optimization and minimax problems using biased estimators.
These methods can potentially be applied to deep learning, statistical learning, generative adversarial nets, and federated learning. This talk is based on the collaboration with Nhan Pham (UNC), Deyi Liu (UNC), Lam M. Nguyen (IBM), and Dzung Phan (IBM).