A Brief Tutorial on Image-based Crowd Counting
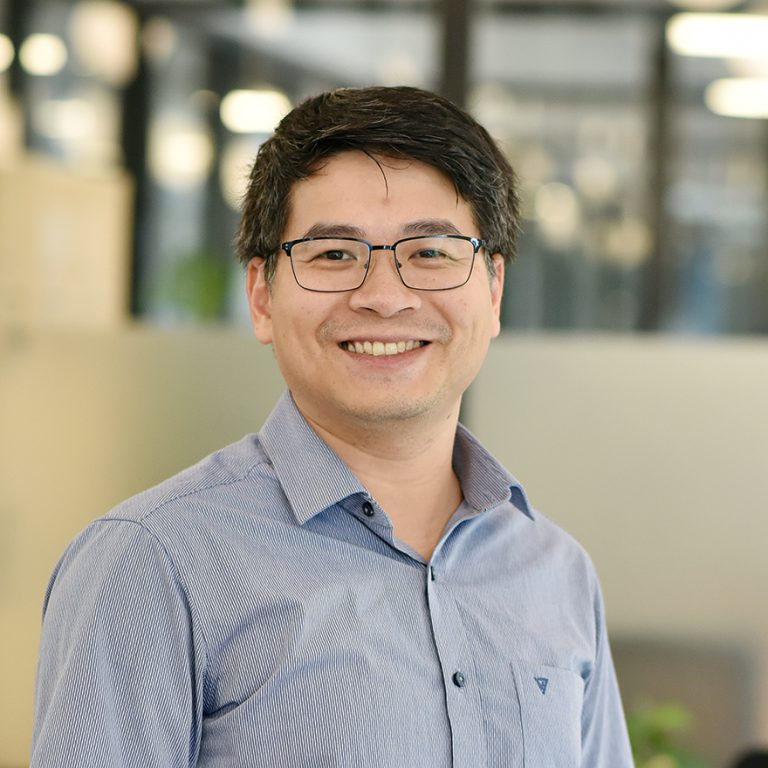
Minh Hoai Nguyen is an Assistant Professor of Computer Science at Stony Brook University. Prior to VinAI and Stony Brook University, he was a junior research fellow at Oxford University, working with Professor Andrew Zisserman. He received a Bachelor of Software Engineering from the University of New South Wales in 2006 and a Ph.D. in Robotics from Carnegie Mellon University in 2012. His research interests are in computer vision and machine learning. He has received several honors including Best Student Paper Award at the 25th IEEE Conference on Computer Vision and Pattern Recognition (CVPR) and Gold Medal at the 41st International Mathematical Olympiad.
Image-based crowd counting is an important computer vision problem with various applications in many domains ranging from journalism and public safety to architecture design and urban planning. In the first part of the tutorial, he will describe the main approaches for both manual and automatic crowd counting. In the second part of the tutorial, we will delve into the details of two recent papers to study their architectures and frameworks. In the third part of the tutorial, He will go beyond crowd counting and describe a class-agnostic counting method. He will provide Google CoLab notebooks for hands-on experiments.