Fairness in Natural Language Processing
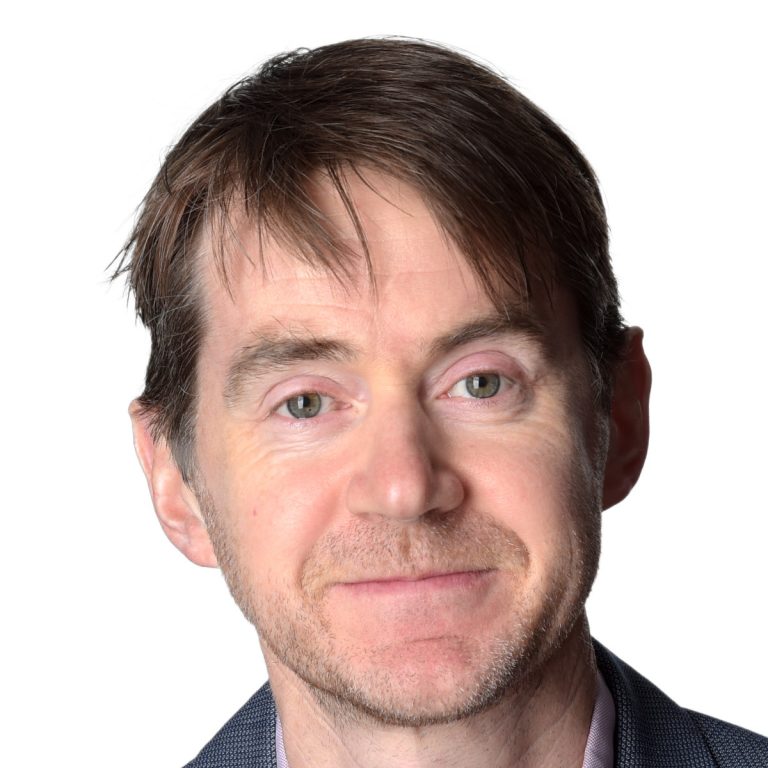
Tim Baldwin is Associate Provost (Academic and Student Affairs) and Head of the Department of Natural Language Processing, Mohamed bin Zayed University of Artificial Intelligence in addition to being a Melbourne Laureate Professor in the School of Computing and Information Systems, The University of Melbourne. His primary research focus is on natural language processing (NLP), including social media analytics, deep learning, and computational social science. Tim completed a BSc (CS/Maths) and BA (Linguistics/Japanese) at The University of Melbourne in 1995, and an MEng (CS) and PhD (CS) at the Tokyo Institute of Technology in 1998 and 2001, respectively. Prior to joining The University of Melbourne in 2004, he was a Senior Research Engineer at the Center for the Study of Language and Information, Stanford University (2001-2004). His research has been funded by organisations including the Australia Research Council, Google, Microsoft, Xerox, ByteDance, SEEK, NTT, and Fujitsu, and has been featured in MIT Tech Review, Wired, IEEE Spectrum, The Times, ABC News, The Age/Sydney Morning Herald, Australian Financial Review, and The Australian. He is the author of over 450 peer-reviewed publications across diverse topics in natural language processing and AI, with over 19,000 citations and an h-index of 66 (Google Scholar), in addition to being an ARC Future Fellow, and the recipient of a number of best paper awards at top conferences.
Natural language processing (NLP) has made truly impressive progress in recent years, and is being deployed in an ever-increasing range of user-facing settings. Accompanied by this progress has been a growing realisation of inequities in the performance of naively-trained NLP models for users of different demographics, with minorities typically experiencing lower performance levels. In this talk, I will illustrate the nature and magnitude of the problem, outline a number of approaches that can be used to train fairer models based on different data settings, but at the same time highlight issues in model selection and evaluation, and the impact of data conditions on the performance of different methods.