Model-constrained deep learning approaches for inference, control, optimization, and uncertainty quantification
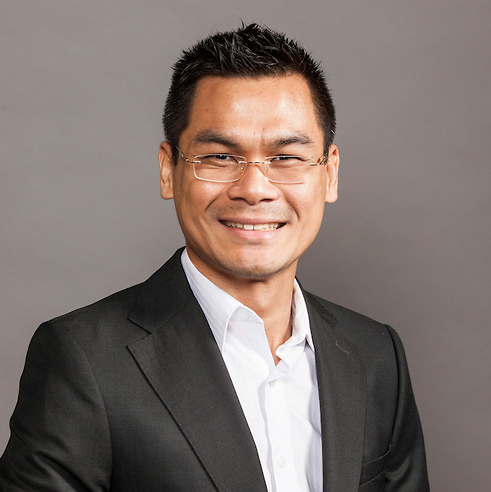
Tan Bui is an associate professor of the Oden Institute for Computational Engineering & Sciences, and the Department of Aerospace Engineering & Engieering mechanics at the university of Texas at Austin. Dr. Bui obtained his PhD from the Massachusetts Institute of Technology in 2007, Master of Sciences from the Singapore MIT-Alliance in 2003, and Bachelor of Engineering from the Ho Chi Minh City University of Technology (DHBK) in 2001. Dr Bui has decades of experience and expertise on multidisciplinary research across the boundaries of different branches of computational science, engineering, and mathematics, and has been plenary/keynote speakers at various international conferences and workshops. Dr. Bui is currently the elected vice president of the SIAM Texas-Louisiana Section, the elected secretary of the SIAM SIAG/CSE, an associate editor of SIAM Journal on Scientific Computing, an editorial board member of the Elsevier Computer & Mathematics with Applications, and an associate editor of the Elsevier Journal of Computational Physics. Dr. Bui was an NSF career recipient, the Oden Institute distinguished research award, and a two-time winner of the Moncrief Faculty Challenging award.
The fast growth in practical applications of machine learning in a range of contexts has fueled a renewed interest in machine learning methods over recent years. Subsequently, scientific machine learning is an emerging discipline which merges scientific computing and machine learning. Whilst scientific computing focuses on large-scale models that are derived from scientific laws describing physical phenomena, machine learning focuses on developing data-driven models which require minimal knowledge and prior assumptions. With the contrast between these two approaches follows different advantages: scientific models are effective at extrapolation and can be fit with small data and few parameters whereas machine learning models require “big data” and a large number of parameters but are not biased by the validity of prior assumptions. Scientific machine learning endeavours to combine the two disciplines in order to develop models that retain the advantages from their respective disciplines. Specifically, it works to develop explainable models that are data-driven but require less data than traditional machine learning methods through the utilization of centuries of scientific literature. The resulting model therefore possesses knowledge that prevents overfitting, reduces the number of parameters, and promotes extrapolatability of the model while still utilizing machine learning techniques to learn the terms that are unexplainable by prior assumptions. We call these hybrid data-driven models as “model-aware machine learning” (MA-ML) methods. In this talk, we present a few efforts in this MA-ML direction: 1) ROM-ML approach, and 2) Autoencoder-based Inversion (AI) approach. Theoretical results for linear PDE-constrained inverse problems and numerical results various nonlinear PDE-constrained inverse problems will be presented to demonstrate the validity of the proposed approaches.