Rumour and Disinformation Detection in Online Conversations
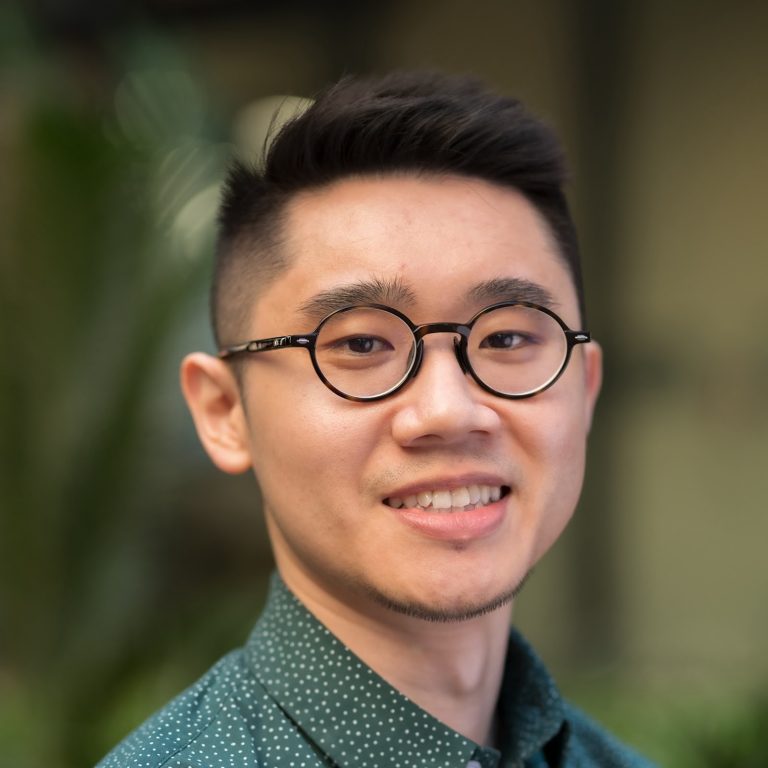
Dr. Lau is a Senior Lecturer in the School of Computing and Information Systems at the University of Melbourne. His research is in Natural Language Processing — a sub-field of Artificial Intelligence — where the goal is to create computational models to understand human languages. A recurring theme of Dr. Lau’s research is the development of computational models in unsupervised or semi-supervised settings, i.e. learning scenarios where the models are trained without access to a significant amount of supervision signal. His research is also characterised by a diverse flavour of applications, such as topic models, lexical semantics, text generation and misinformation detection. Some of his work has generated broader community interest beyond academia, e.g. his research in poetry generation and influence operations has been featured in science magazines (New Scientist) and mainstream news media (Guardian and BBC), and his latest collaboration with an artist to develop a personality-mimicking dating chatbot is currently being showcased at NGV Australia.
In the first part of the talk, I’ll discuss a method for detecting rumors by analyzing online conversations. Motivated by the observation that the user network — which reveals *who* engage with a story — and the comment network — which shows *how* they react to it — provide complementary signals for rumour detection, we show how transformer and graph attention networks can be used to jointly model the content and structure of social media conversations. Moving to the second part of the talk, I’ll introduce another project focused on identifying state-sponsored agents involved in disinformation campaigns. Existing detection systems are developed based on training data for known campaigns, and they fall short when dealing with new campaigns. Here I’ll demonstrate how a combination of meta-learning and transformer adapters can address this shortcoming, enabling us to detect emerging campaigns in different languages as they evolve over time.