Statistical Rule Learning for Materials Science
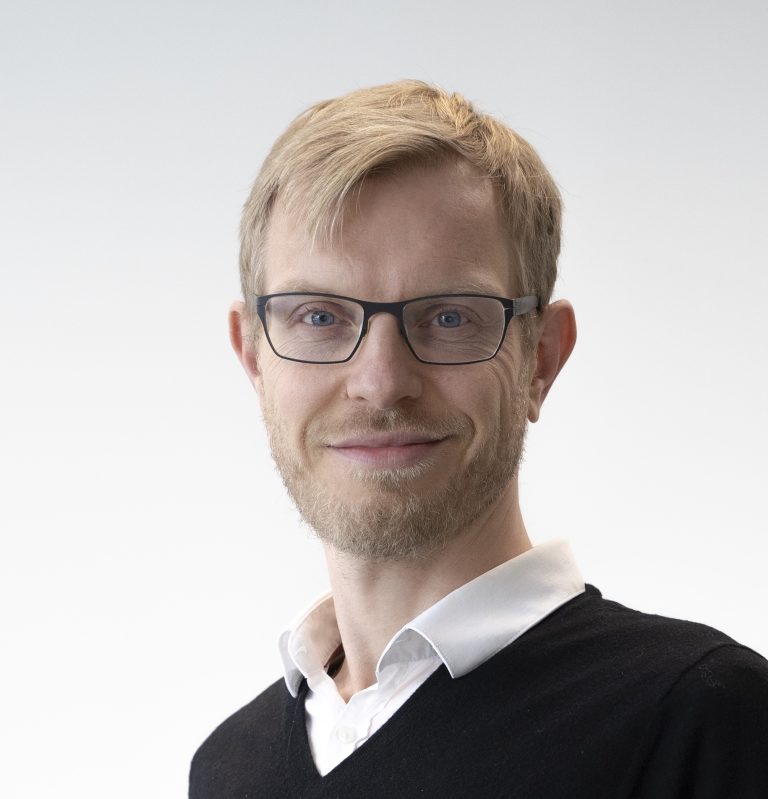
Mario Boley is a senior lecturer in the Department of Data Science and AI at the Faculty of Information Technology at Monash. His research is on interpretable modelling and data-driven knowledge discovery where he works on theoretical algorithmic questions as well as on interdisciplinary applications. Specifically, he is interest in machine learning models for the discovery of novel materials – a topic he first came in touch with as investigator in the European NOMAD Centre of Excellence in 2015. His background is in computer science where he obtained his Ph.D. in 2011 in Bonn, Germany, for work in algorithmic order theory.
Despite notable progress in the application of machine learning to materials science, the opaque form and unsatisfactory extrapolation performance of current models precludes the discovery of novel materials or general scientific insights. Improved ML models are therefore actively researched, but their design is currently guided mainly by monitoring the average in-distribution test error. Unfortunately, this can render different models indistinguishable although their performance differs substantially across individual materials. Moreover, a sole focus on predictive performance neglects the syntactic form of the model and disregards physical knowledge that could be used to assess whether the model is likely to generalise outside of the training distribution. Here, we discuss how rule-based models can be applied to alleviate both problems. Firstly, they can be used to detect and describe the domains of applicability (DA) of complex models within a materials class. Secondly, they can directly be employed to model properties of the materials of interest. In both cases, they provide an interpretable machine learning layer to materials modelling that can drive the formulation of hypotheses and targeted acquisition of further training data.