Ubiquitous 3D Vision in the Wild
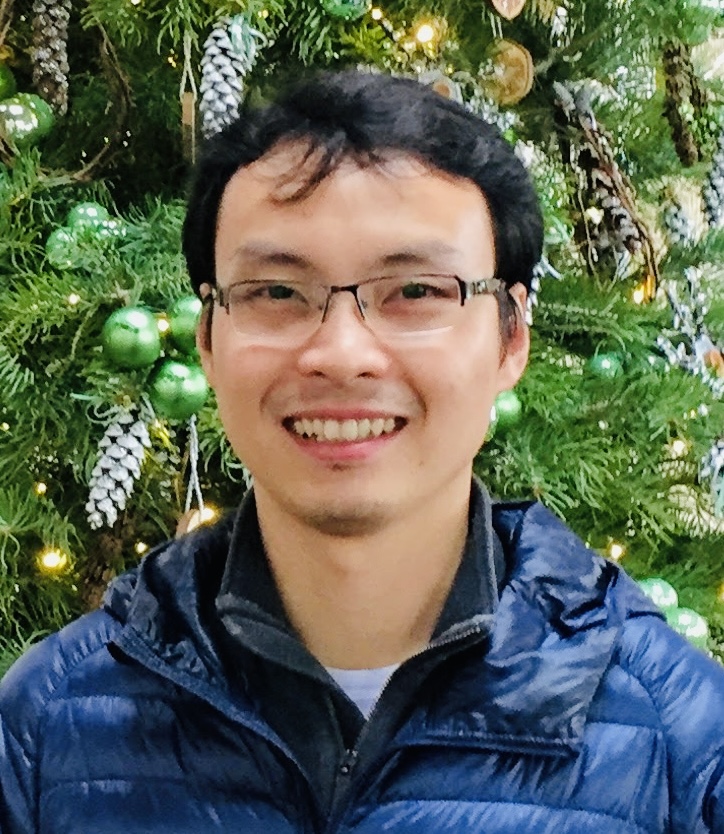
Minh Vo is the Head of Machine Learning at Spree3D, a high-tech virtual try-on startup. Minh leads a team of passionate researchers and engineers to strategically develop and commercialize our photorealistic avatar technology. Previously, Minh was a Senior Research Scientist at Facebook Reality Labs Research, where he tech-led a group of researchers to develop 3D perception and human sensing algorithms for Meta Aria glasses. Minh got his Ph.D. from The Robotics Institute, Carnegie Mellon University, where he worked with Prof. Srinivasa Narasimhan and Prof. Yaser Sheikh on novel methods to capture dense and accurate 3D shape of human bodies. His Ph.D. work was awarded the prestigious Qualcomm Innovation 2018 Fellowship.
As cameras become ubiquitous, there is an increasing opportunity to reliably detect, reconstruct, and track in 3D the visual data in those footages for downstream applications, like surveillance, user intent understanding, or creative purposes. In this talk, we discuss our recent progress in 3D object scene understanding from three different platforms: a single infrastructure camera, a single wearable smart glasses, and collections of data captured by smartphone cameras. Firstly, we present Snipper, a novel framework to jointly detect, track, and forecast future human motion from an RGB video snippet. Despite its simplicity, Snipper outperforms many existing methods in stationary infrastructure camera tracking settings. Secondly, we present AriaHuman, the first large-scale 3D multi-human tracking benchmark acquired under different environments and activities from a smart glass setting, and a baseline method that takes advantage of the multiple cameras stream commonly available in the glass settings. Finally, we present BANMo, the first method to create 3D textured shape and estimate the motion of humans and animals in a unified manner from many casual videos that were not captured simultaneously.