(Un)trustworthy Machine Learning: How to Balance Security, Accuracy, and Privacy
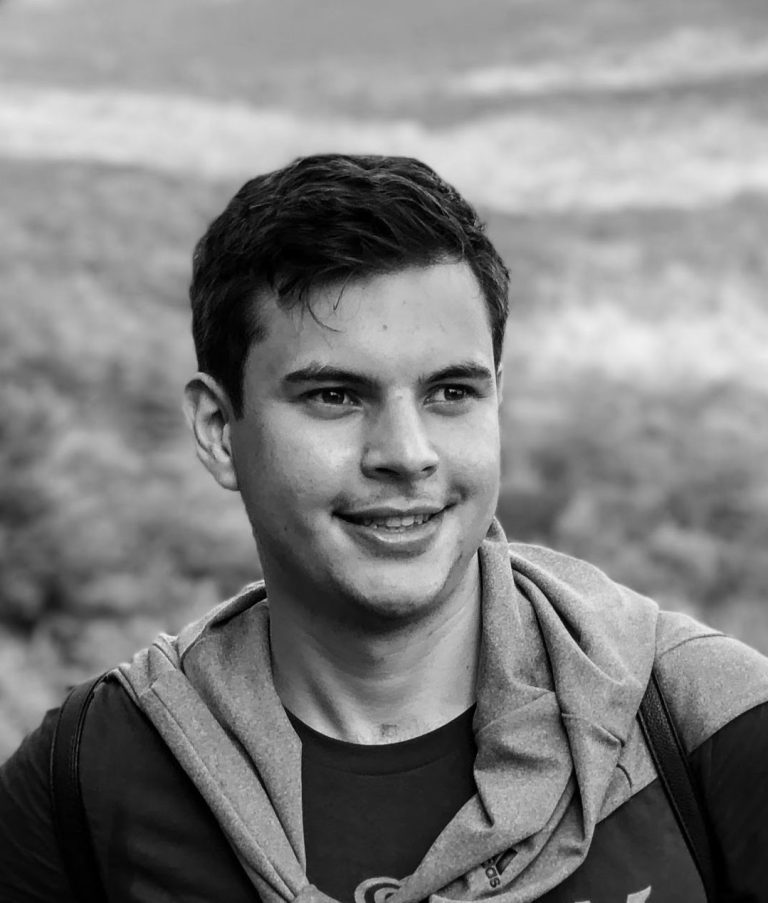
Eugene Bagdasaryan is a doctoral candidate at Cornell University, where he is advised by Vitaly Shmatikov and Deborah Estrin. He studies how machine learning systems can fail or cause harm and how to make these systems better. His research has been published at security and privacy and machine learning venues and has been recognized by the Apple Scholars in AI/ML PhD fellowship.
Machine learning methods have become a commodity in the toolkits of both researchers and practitioners. For performance and privacy reasons, new applications often rely on third-party code or pretrained models, train on crowd-sourced data, and sometimes move learning to users’ devices. This introduces vulnerabilities such as backdoors, i.e., unrelated tasks that the model may unintentionally learn when an adversary controls parts of the training data or pipeline. In this talk, he will identify new threats to ML models and propose approaches that balance security, accuracy, and privacy without disruptive changes to the existing training infrastructures.